This audio was created using Microsoft Azure Speech Services
by Claude Le Pape-Gardeux and Jacques Kluska
The surge in AI technology is raising questions about its electricity usage and related GHG emissions. According to International Energy Agency (IEA), data centers, AI and cryptocurrency are responsible for 2% of total global electricity demand. However, IEA predicts that by 2026, the AI industry alone is expected to have grown exponentially to consume at least ten times its demand in 2023. As AI technology continues to evolve at an exponential pace, it is imperative to measure and limit its environmental impact to keep this energy consumption at the lowest level possible.
At Schneider Electric, since the opening of our AI Hub, the strategic objective was to apply AI to the biggest challenges of our time, such as climate change, and to support our customers in their sustainability journeys. Putting AI to work to achieve substantial energy efficiency gains requires us to monitor the other side of the equation – how much energy and resources the AI systems consume themselves. As our AI experts were developing methods to assess the efficiency of AI systems, we saw a clear need to establish global standards in this field.
How to design frugal AI systems?
In January 2024 we have joined forces with the French standardization body, AFNOR, that took the initiative to develop an “AFNOR Spec on Frugal AI”, aimed at defining best practices and encouraging adherence to environmentally responsible AI standards.
We are strongly promoting a frugal approach to AI development which consists of moderation by design, ongoing search for efficiency, and proper balance between the environmental value and cost.
While working on the guidelines with other contributors, our primary objective was to comprehensively address the environmental impact of AI while providing practical guidance that organizations and individuals can readily implement when designing and operating AI systems.
The collaborative effort involved 140 participants representing a diverse array of organizations, including industry, startups, public sector, NGOs, and academia. The three working groups, focusing on “definitions and communication”, “environmental indicators and methodology”, and “best practices”, worked tirelessly to ensure the document’s relevance and practicality.
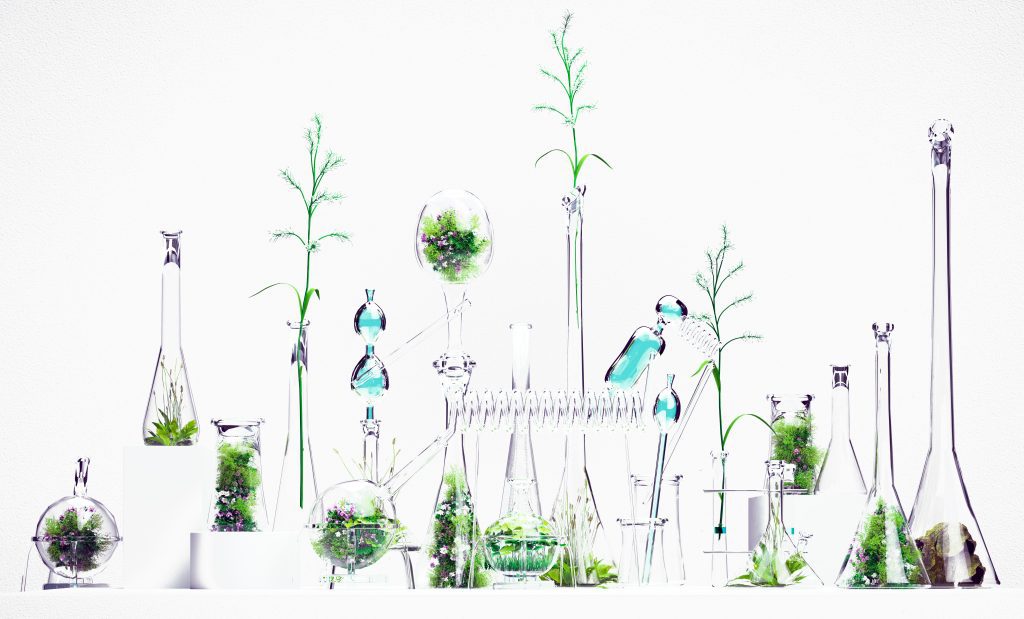
Looking for frugal consensus
It was truly rewarding to exchange ideas with experts and professionals from various domains. One of the most intense debates was about the scope of frugality assessment. For an AI system serving to improve energy efficiency or reduce carbon emissions, we can compare two figures:
1) the amount of carbon saved by the AI-enabled solution, and
2) the amount of carbon emitted while developing and running the solution. Obviously, the first figure should be considerably higher than the second.
However, it is disputable how to weigh both sides if the AI solution doesn’t have a direct effect on carbon emission decrease, but is used in a completely different field, like healthcare or finance. Thus, three definitions emerged:
- “efficient AI systems” highlighting how we can optimize an AI system (the model, data, etc.) to minimize its environmental impact,
- “frugal AI services”, considering the whole service chain and usages of the service,
- “AI services with positive impact” along a given category of impact (e.g., carbon emissions, water consumption, etc.), when it is shown that, for this category, the positive impacts of the service exceed the negative impacts.
Using our experience from Schneider Electric AI projects, we contributed insights tailored to industry-specific AI applications, offering guidance on measuring impact and defining the concept of frugality within the context of AI services. We also gained valuable insights and fortified our frugal AI assessment methodology.
Three questions to ensure frugality of AI
The key to creating frugal AI solutions is to focus on these three questions:
1. Do we need to apply AI in the first place?
We should use AI to solve problems. Some AI solutions intend to save energy or carbon emissions, some are used for other purposes, saving time or cost, improving system reliability or safety, etc. In both cases, it is crucial to check whether other methods exist, and whether AI is more effective than other methods.
2. How can we use AI as efficiently as possible?
The starting point is to measure the performance of AI, considering the results and the related costs. If the goal of an AI solution is to save energy or environmental emissions, then we simply need to include the energy/CO2 cost of AI in the evaluation. If AI is used for another purpose, e.g., safety, then we need to evaluate the compromise between energy and CO2 cost and improved safety.
3. How to improve the AI use carbon-wise?
Even if the overall balance is satisfactory, there is always room for improvement.
This can be achieved in several ways:
(a) use the AI-based application less frequently, e.g., revise energy production/consumption forecasts once versus four times an hour,
(b) use green energy to run the AI computations,
(c) improve the solution architecture, e.g., make more computations on edge, use more energy-efficient hardware, etc.,
(d) make tradeoffs on model precision, e.g., smaller data sets, smaller neural networks, parameters coded with 8 bits rather than 64,
(e) inject knowledge in a machine learning procedure, e.g., using physics-informed neural networks,
(f) use a hybrid method, e.g., AI for forecasting combined with linear optimization for planning.
Some of these methods have a potential impact on solution quality, so we need to review the compromises between carbon footprint and the performance of the solution.
Efficient AI solutions as an industry benchmark
The AFNOR Spec on Frugal AI, published in June 2024, represents a significant milestone in the journey towards establishing international standards for environmentally conscious AI practices. This collaborative endeavor has not only enriched our approach to AI but also reinforced the commitment of all contributors to driving sustainable innovation.
As mentioned by our Chief AI Officer, Philippe Rambach: “We are passionate about efficiency and we want to encourage others to align with the highest standards of environmental responsibility of AI systems and services.” Our active participation in shaping Frugal AI standards exemplifies our dedication to advancing technology in harmony with environmental sustainability.
Interested to learn more?
- Read the announcement and access the AFNOR Spec on Frugal AI to read full publication.
- Listen to “Frugal AI. The other side of the equation” AI at scale Podcast episode with Claude Le Pape-Gardeux.
About the author
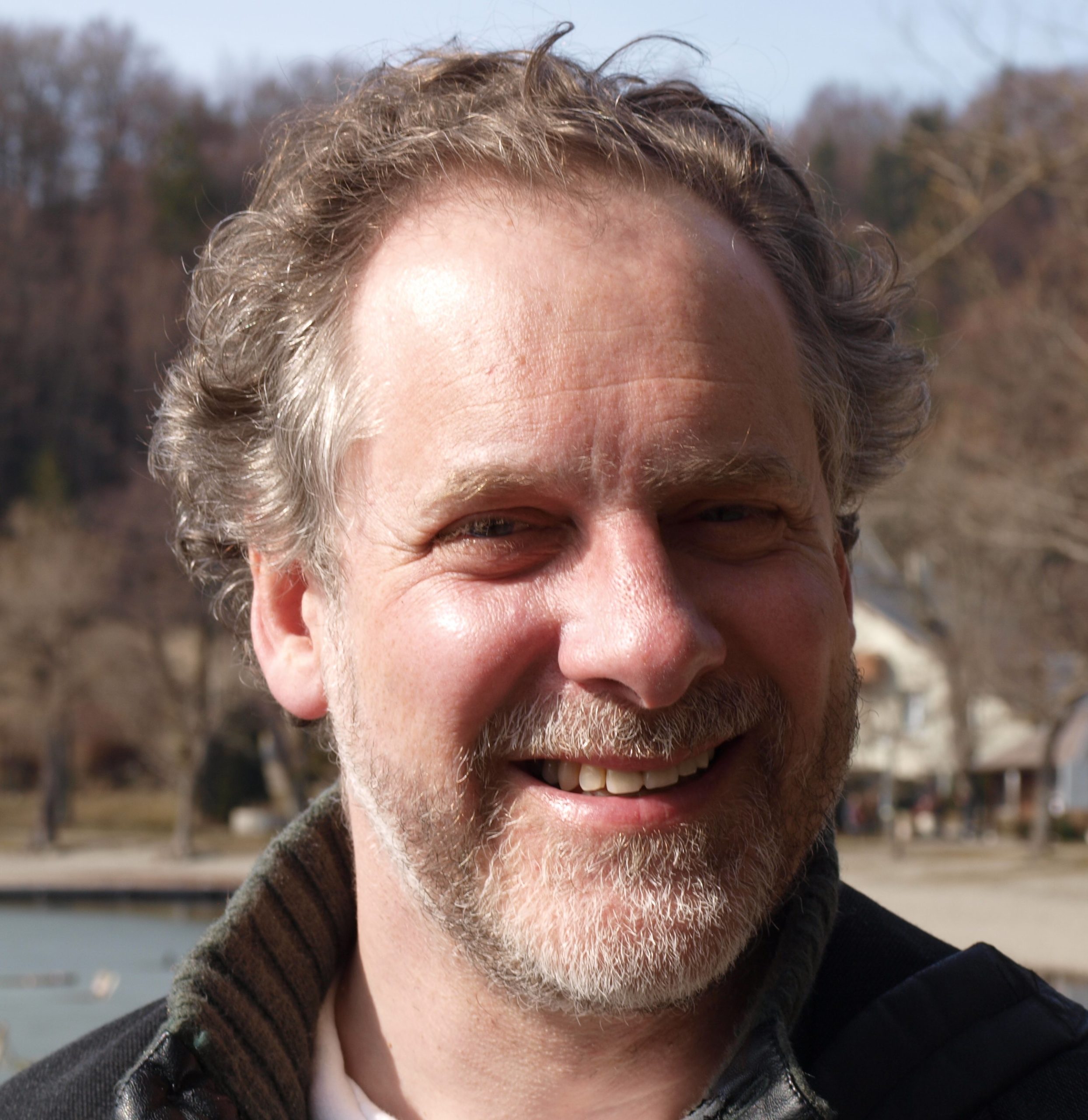
Claude Le Pape-Gardeux – Data & AI Domain Leader
Claude Le Pape is coordinating the evaluation of new technologies, the recognition of technical experts, and the management of Research and Development projects and partnerships in the Data and Artificial Intelligence domain in Schneider Electric. He received a PhD in Computer Science from University Paris XI and a Management Degree from “Collège des Ingénieurs” in 1988. From 1989 to 2007, he was successively postdoctoral student at Stanford University, consultant and software developer at ILOG S.A., senior researcher at Bouygues S.A., and R&D team leader at Bouygues Telecom and ILOG S.A.
He contributed to several European research projects and to the development of many software tools and applications in various domains: chemicals mixture design, inventory management, manufacturing scheduling, long-term personnel planning, construction site scheduling, and energy usage optimization. He is member of the Scientific Advisory Board of “Institut Mines-Télécom” and of the French National Academy of Technology.
About the author
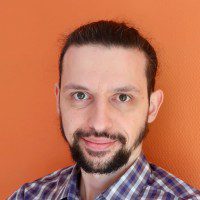
Jacques Kluska – Expert Data Scientist
As an expert data scientist at the AI Hub, Jacques Kluska brought over a decade of AI experience from his work as an astrophysicist before joining Schneider Electric in April 2023. Having obtained a PhD in 2014 focusing on image generation for astrophysics, Jacques has been instrumental in developing AI solutions utilizing computer vision, timeseries, and generative AI for various applications, including predictive maintenance, anomaly detection, and generative design. Currently, he is actively engaged in advancing Sustainable AI initiatives from both normative and operational perspectives.
Add a comment