AI at Scale podcast is live!
The first Schneider Electric podcast dedicated only to artificial intelligence is officially live and available on all streaming platforms. The AI at Scale podcast invites AI practitioners and AI experts to share their experiences, insights, and AI success stories. Through casual conversations, the show provides answers to questions such as: How do I implement AI successfully and sustainably? How do I make a real impact with AI? The show features real AI solutions and innovations and offers a sneak peek into the future.
Some of the most difficult questions that a Chief AI Officer can get

The first episode hosts Philippe Rambach, Schneider Electric’s Chief AI Officer. In the conversation, Philippe answers some of the most difficult questions that a Chief AI Officer can receive. He explains when it is necessary to use AI and when not, the sustainable and frugal approach to AI, and finally, talks about three pillars of AI supporting decarbonization and energy savings. Then, in the second part of the show, Philippe shares practical insights for businesses about implementing AI at scale in the company to ensure return on investment and value from AI.
Listen to the first episode of the AI at Scale podcast
Listen to Philippe Rambach: AI at SCALE. How? When? And why? episode. Subscribe to the show on the preferred streaming platform (Spotify, Apple Podcasts), listen to the episode on YouTube, and stay tuned for the next episode.
Transcript
Narrator: Welcome to the AI at Scale podcast. This is a show that invites AI practitioners and AI experts to share their experiences, challenges, and AI success stories. These conversations will provide answers to your “How do I implement AI successfully and sustainably? How do I make a real impact with AI?” Our podcast features real AI solutions and innovations, all of them ready for you to harness and offer a sneak peek into the future.
Gosia Górska: Hi, I’m Gosia Górska, and I’m the host of the Schneider Electric AI at Scale podcast. I’m pleased to introduce my first guest, Philippe Rambach, SVP and Chief AI Officer at Schneider Electric. Philippe is a graduate of École Polytechnique in France and joined Schneider in 2010 from Areva. He has more than 20 years of experience in strategy, innovation, and business responsibility in many industries. He held various leadership roles in energy management and industrial automation, most recently as SVP of the Industrial Automation Commercial Unit. He has an international career background and is currently based in France. Welcome, Philippe. Thanks for joining the show.
Philippe: Hey Gosia, thank you for having me.
The role and mission of the Chief AI Officer
Gosia: This is our first episode of the AI at Scale podcast, and it couldn’t start differently but with a person who was appointed as the first Chief AI Officer of Schneider Electric in November 2021. Now, I collected a list of the most difficult questions that a Chief AI Officer can get, so I hope you are ready. But let’s start with explaining what’s your mission as Chief AI Officer in the company?
Philippe: Yeah, sure, I’m ready. As you said, I’m the first Chief AI Officer, and that’s a journey that we started two years ago. And the idea was, we believe it is time now to take AI out of the labs, take AI out of the innovation centers, take AI out of the MVPs, and really bring AI at scale. So my job is how do we make sure that in Schneider, we leverage AI at scale with a real impact, both on the way we work, our internal efficiency, the support of our customers, and how we can also help our customers be more sustainable, leveraging AI in our offers. So really a transformation job where we don’t want to take a technical approach, but a use case approach to bring AI at scale for the benefit of customers and employees.
AI’s potential and misconceptions
Gosia: And if we start with more difficult questions. So you mentioned a bit what’s the role of AI for Schneider Electric and what’s your mission, but if we think overall about other companies, why do we need AI in the first place? Why use a technology that, especially today, we hear comes with so many controversies around cybersecurity risks, ethical considerations, and potential harmful applications?
Philippe: So first, I would say, if you can achieve your goals without AI, don’t use AI. However, I think that AI is today an incredible tool to help you achieve your goals. And I think the moment is now, because we have reached a time where a lot of investments have been done in data, in cloud, in compute power. There are also new algorithms coming, like Generative AI, which makes it even easier to use. So it’s really the right time to take AI, to use it at scale, to improve customer satisfaction, customer offers, and employees.
Then I think also that quite often, when people think about AI, they think about mind manipulation, social scoring, disinformation. But people forget that most of AI is actually used for very good things. Typically in what we do, we use AI to reduce our impact on the planet. We use AI to optimize the way we use energy, to use more green energy, to reduce waste, etc. And we don’t touch a lot of personal information. We mostly manipulate machine information, electrical information—nothing personal, nothing about people.
Gosia: So there is indeed a huge potential in artificial intelligence and the way that we can tackle large amounts of data coming from building sensors, for example, or industrial processes that are very energy-intensive. And probably this is really the technology that we’ve been waiting for to optimize all of this. What about carbon footprint, energy consumption, and water usage of AI models? There is a growing concern around the influence of AI on the environment. What’s your point of view here?
Environmental Impact of AI
Philippe: I think that’s a real question, and we need to really be careful and pay attention to that. And I would say that two answers. The first thing is, when we use AI or when we develop AI models in order to be more sustainable, we need to check that it works, that the investments we do in terms of carbon to run the model—because there is an investment—is matched by the savings, big time. And today we are at the very early stages of that, and actually the savings are in the range of 100 to 1000 times more than the investment. But we need to think ROI, carbon ROI: How much carbon do I invest? How much carbon do I save?
I take a basic example, like you mentioned, building operations. So to use AI-based algorithms to optimize energy management in a building, it’s going to cost like 0.003 ton of CO2 per year in additional compute. And for an average-sized building, we can save two tons of CO2. So the ratio is probably one to 600. Or if I take a more ambitious example, where we optimize a full microgrid at the level of a university or at the level of a big shopping mall, running the model will be slightly more expensive, probably 0.1 ton of CO2 per year, but the savings will go from 300 tons to 500 tons. So a ratio of one to 3000 or one to 5000.
So yes, we do more savings than cost if we use AI for good. This being said, if you use AI to choose your next Netflix movie, you may have zero impact on the planet and a lot of cost of carbon, but a lot of applications of AI really help the planet. But again, you’re right, you need to look at the cost of carbon and the return.
The second thing is, what I like with data centers is they are powered with electricity. And we also have a lot of efforts with data centers, with our customers, to work on how we can help those data centers use as much as possible of green electricity to reduce the carbon footprint of running those models. So working both on the impact and on the cost of running the models.
Gosia: And I guess also people tend to think about Generative AI as a tool, a solution to every challenge that we are facing, and especially ChatGPT is actually problematic because the cost of the energy that it consumes is considerably higher than other AI techniques, right? What’s your point here?
Philippe: Yes, that’s super important. And we should not be blinded by the beauty of the newest, latest technology. If I look at how we can use AI to reduce the impact on the planet, most of it is actually about forecasting, anomaly detection, optimization. And for that, large language models are not the best solution. And we have much more frugal AI techniques, which cost much less. We don’t need hundreds of billions of parameters. We can do the job, and sometimes much better than an LLM or a Generative AI.
So my first recommendation is choose the right model, the most frugal model, not the newest model. And then even if you sometimes need Generative AI, of course, make sure that you use the right size model. Sometimes some small language models can do the job as good as large language models. Sometimes you don’t need GPT-4, but GPT-3.5 can make the job. If I look at what we do internally in Schneider for our customers using LLMs, we stayed with 3.5 because the carbon impact is much lower than 4.0.
AI applications in energy optimization
Gosia: Exactly. And when you discuss these opportunities with customers, what are the biggest opportunities for them? What are the areas in terms of reduction of carbon emissions and optimization that are solved by AI?
Philippe: Yeah, I would say when I think about AI and decarbonization, energy saving, I would say three pillars. The first one is simply do the same with less—use less energy for the same outcome. And here, AI can play a very strong role in optimizing your processes. When you’re not able to build a complete physical model of your process to optimize it, you can use AI, use machine learning to build an AI-based model, and use that model for optimization. Typically, that can be used to save energy in buildings, in factories.
Then of course, it’s always good to use less energy, because if you use less energy, you generate less carbon. However, the only energy that can be fully decarbonized is electricity. So you also need to move to electricity. And here, AI has a role to play in two domains. The first one is the electrical grid with renewables is much more complicated than a grid without renewables, and AI will be needed to optimize and operate this grid.
The second topic is when people think about having green electricity, they mostly think about the production side. They think about installing solar panels, wind generation, and that is good and that is needed. But we also need to work on the demand side.AI here can really help averaging the demand, avoid the peak of demand. As you know, when there is a peak of demand on the electrical grid, then the electricity is usually quite carbon-intensive. And anything that can help averaging the demand will help using more green electricity. So AI here will help planning and forecasting and optimizing to average the demand.
The third pillar, which people very often forget, is if we have great AI-based solutions or non-AI-based solutions to optimize energy and use less energy—even if we have good solutions to electrify everything—we still need to do that at scale. If we only use these solutions on one or two or three buildings in the world, we haven’t moved the needle. We need to use them all across the world. AI here again can play a very strong role in removing the barrier to adoption, making it much faster, much easier to deploy.
I’ll take a very quick example of something we have done in California. California wants to move fast to have solar panels on every individual home. The first step of that is to have an electrician coming to your home to check your installation. There are not enough electricians in California—that is slowing the deployment. We have developed an AI module where you open your electrical panel, take a picture, and automatically get a recommendation of whether you can or cannot install a solar panel. So in short: optimizing processes to save energy, AI helping to move to electricity, and then AI helping to do that at scale.
Gosia: Yeah. So these are definitely the three pillars for any company to start thinking about implementing these AI solutions. And when we think about it from the practical point, what should be the starting point of implementing these solutions? How easy or difficult is it to implement them in a company’s building or production process?
Implementing AI solutions: challenges and starting points
Philippe: I would say the starting point needs to be a business need, an employee need, a customer need. Starting point is not “What can I do with AI?” but “What are my problems and what are the ones that AI can solve?” That’s the starting point. Then of course you need to have invested a bit in your data. There is no AI without data.
I would also like to emphasize the importance of the acculturation, knowledge of your employees. Not everybody needs to be an in-depth data scientist, but people need to understand enough of AI to avoid either being in the group of people who say, “Yeah, it will solve everything,” or the people who say, “You know what, we had metaverse, we had bitcoin, we had blockchain. Let’s wait a bit; it’s going to go away.” It will not go away.
Gosia: Exactly. So this kind of understanding of the potential but also about the limits of AI is probably crucial here. You mentioned about the investment… What’s the level of investment needed?
Philippe: I would say for many, many companies, they have done most of the investment. The big cost was collecting the data, organizing the cloud, organizing the data. The additional cost to run AI is not that big. We are moving from cost to benefit, then we move from investments to reaping the investment by putting AI on top of it. I cannot give a number, of course; it’s very company-dependent. But if you look at it as a company, you realize that you have spent already most of what you need to do AI, and you just miss now the top to really get the outcome of your investments in data, cloud, and so on.
Gosia: I see. What kind of results can the average company expect? What is the main benefit that you see—the immediate one?
Philippe: Of course there are immediate benefits about using AI to increase your internal efficiency, to increase your customer satisfaction. But I would like to focus on, as you know, here from the beginning, what is important for me: climate change. By implementing AI solutions in regards to optimizing your energy—I mean, take a few examples of customers. Citycon, one of our customers in the Nordics, have reduced simply by optimizing their energy using some solutions more than 300 tons of CO2 every year—direct energy savings using our machine learning algorithm to analyze data and help them always optimize their choice between using what their renewable is produced, buying from the grid, selling to the grid, storing the battery, etcetera. So that was a typical example. So it’s a lot of carbon savings.
Another example in the same kind of range is in Croatia. We have deployed a solution to central district heating system with our AI, where we have seen massive carbon savings.
Gosia: And I think it’s also clarifying the fact that we don’t have to wait anymore for a magic technology to come to solve climate change issues. We actually have a technology that is very powerful; we just need to know how to use it and that this kind of benefits that you just mentioned are actually available to all of the companies, all of the organizations.
Philippe: Yeah, sometimes it’s a bit upsetting. I mean, we do have the solutions to massive problems; we now need to adopt and deploy.
Scaling and adoption of AI solutions
Gosia: Overall, around 70% of digital transformation projects fail. Two main obstacles that companies fight with are scaling and adoption. Philippe, what’s your secret sauce for scaling AI solutions?
Philippe: First, it is difficult, but what we have learned is first start from the business case, start from business value, never start from the technology. And the second key point is in regards to AI, because it’s new, many people actually have two processes. They have a first process or team to go from idea to proof of concept or minimum viable product, and then another team will industrialize. Guess what? Industrialization never happens. I really believe that now AI is mature enough that we have processes that go directly with the same team from ideation to operation. Of course, in between you will do MVP and proof of concept, but start with the team that has the mission to deliver the solution at scale, not to deliver a proof of concept or an MVP.
Gosia: And what’s your point of view about using off-the-shelf solutions and solutions that need to be created specifically for a company, like bespoke AI solutions?
Philippe: Yeah, I would say that it’s not really an AI-specific question. It’s a usual make-or-buy in the IT world. My recommendation is always, if you can buy, buy. Make is always difficult, takes time. If you can buy, buy—except of course if it’s in your core business. In AI, still a lot of things need to be built, because still a lot of things are a bit data-specific, solution-specific, etcetera. It’s still a business where you need to make a lot of make. The coming of Generative AI makes it easier also to go to much more off-the-shelf products.
Gosia: We mentioned at the beginning that your mission was to establish the whole organization dedicated to artificial intelligence at Schneider. So how did you tackle this from the scaling perspective, the challenge is how can you merge AI knowledge with domain knowledge? Because the key value comes when you are able to merge it.
Philippe: Of course, it’s very difficult to hire domain knowledge with a lot of AI knowledge. At least these days you can’t find those people. What we’ve decided to do is to use a hub-and-spoke model where on one side we created a strong team of AI specialists. Then when we have identified a business case, a use case where we want to apply AI, we create, in the pure sense of agile, a scrum team, merging people from the AI hub with the AI knowledge, with people from what we call line of businesses or functions like HR, global supply chain, etc. That scrum team made of AI specialists and domain knowledge people deliver the full solution at scale. By doing that, you don’t fully solve the adoption issues, but you make it much easier. You also have a way to solve this issue of having both AI and domain knowledge.
Gosia: Yes, that’s what I wanted to comment when you mentioned that you also invite people from the business teams to be part of the team who is actually working on a specific application. It means that they can bring their domain knowledge. They can bring the real value for the customer that they are aware about. It also means that they will be more open to use the final application in their own business or for the customers, right?
Philippe: Yeah.
Gosia: Is there any other secrets to improve the adoption? You also mentioned that at Schneider we have a lot of applications that are aimed at our own productivity.
Philippe: I would say two things. The first thing is probably don’t try to bring a brand-new AI product because you’re going to need to manage adoption, go-to-market. In many cases, AI will improve existing offers where you already have customers, go-to-market. Focus maybe more on AI features to improve offers than brand-new AI offers. The second thing I would say is never forget that AI is not that different from any other transformation. You will face the same difficulties, the same challenges of employee adoption, employee training, customer adoption, marketing. Sometimes because it’s AI, people think it’s different. No, at the end you have to manage the adoption of a new technology by people, and that takes what it takes. My last secret sauce is, yeah, this is transformation. Manage it as a transformation.
Preparing employees for an AI-embedded world
Gosia: In this case, change management is actually on the top of the key factors to succeed with this transformation. You actually mentioned about preparing employees also for this transformation and I wanted to ask you about how should a company, or overall every one of us, prepare for the world where AI is embedded into most of the tools that we are using in our life?
Philippe: Yeah, I think it is super important in a given company that every employee has some understanding of AI. Of course you don’t need to have the same understanding of AI for every kind of people, but I think you should leave nobody behind. The way we did it at Schneider, for example, is we have classified our employees in four broad categories, from anyone till AI specialists. We have specific trainings for each of them to make sure that everybody understands enough of AI. The good thing is people are curious of AI, so it’s a good opportunity to show them what we do, what they want to learn. It’s easier than other things; people are curious about it.
Gosia: Okay, I have one or two last questions. First, I have the impression that you like your work. Is there any key to make your job well?
The future of AI
Philippe: Good question. I would say probably it’s important to know your business because it’s so easy to be excited with new AI techniques, build shiny objects, proof of concepts, make press releases, when it’s important to work on what will really impact your business. Know your business, know your customers. The second thing I would say is know your company. It is a big transformation. It is a complicated transformation. Every company is different. You need to understand what are the levers you can use, what is the right organization for your company. Then of course, you need some passion for technology, you need to have the passion to learn. It is changing every day. You need to have some kind of, let’s say, enough scientific background to be able to understand what happens in this world, what is smoke and mirrors, and what is really true. That’s what it takes. I think: business knowledge, company understanding, and some passion for science.
Gosia: And do you have any prediction for the future of artificial intelligence?
Philippe: Yeah, that’s a question I cannot answer. Who can predict the future of AI? The only thing I can say is I don’t believe that we are getting in any way near a human general intelligence. What we do is still super specialized. However, in many domains, AI is becoming better than humans at specialized tasks, and that will definitively impact strongly the way we work. It’s a change which is similar scale—not more, not less—than when Internet came, when the personal computer came. I think we are talking about this kind of at-scale change.
Gosia: Okay, so definitely it’s worth to use this tool and also keep in mind that it’s just a tool. On one side, we need to get familiar with it and know how to use it to the advantage of ourselves, the society, and the planet. Thank you, Philippe, thank you. Thank you for coming to the show, and it was a pleasure to host you today.
Philippe: Same here.
Interested in learning more?
- Visit our website to discover how do we transform energy management and industrial automation with artificial intelligence technologies to create more sustainable solutions.
- Read how different AI solutions support sustainability in the blog by Philippe Rambach.
- Listen to the previous episodes of the AI at Scale podcast.
- Read more about AI for energy transition in this e-guide.
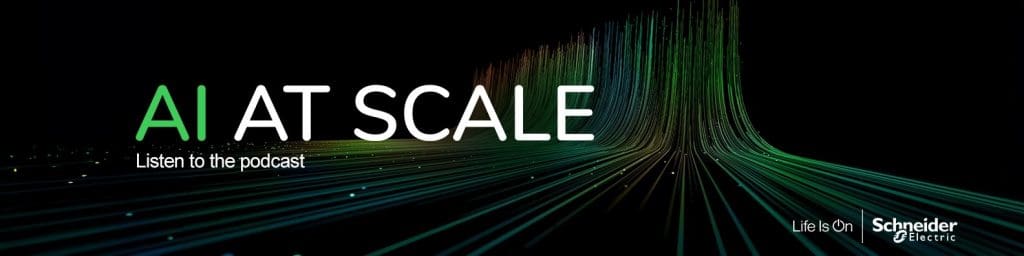
Add a comment