Disruptive power of AI
How exactly do we innovate with AI? How to make strategic bets on new AI-enabled solutions when the technology itself changes so fast? In this episode of the AI at Scale podcast, Gosia Gorska meets an expert from the Schneider Electric AI Strategy and Innovation team – Julia Peyre.
Julia experienced working on AI projects in start-ups and mature organizations. She shares her unique perspective on AI innovation, what it means, and how to innovate with AI in practice.
She unveils the strategic decision-making process between developing AI solutions in-house versus finding ready-to-use, suitable AI products. Furthermore, she shares how she balances these approaches.
In the conversation we also touch on the different types of cooperation, comparing the dynamics of working with start-ups versus the biggest players in the AI field.
Finally, we examine the key criteria that Julia considers when evaluating new AI solutions and the methods she uses to test new AI technologies.
As we publish this episode, Julia has been appointed as the Head of AI Strategy and Innovation of Schneider Electric. Tune in to our conversation to gain invaluable insights from our AI leadership team!
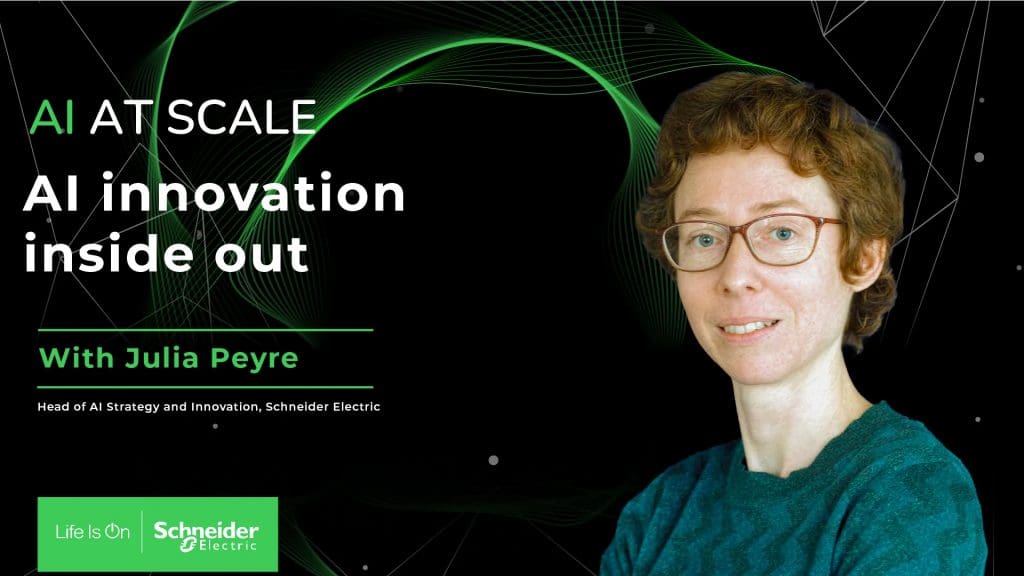
Listen to the AI at Scale podcast
Listen to Julia Peyre: AI Innovation Inside Out episode.
Subscribe to the show on the preferred streaming platform (Apple Podcasts, Spotify) or play the episode on youtube.
Transcript
Gosia Gorska: Hi, I’m Gosia Gorska and I’m the host of the Schneider Electric AI Scale podcast. Today, I have the pleasure to talk with Julia Peyre. Julia is a graduate of École Polytechnique with a PhD in Computer Vision from Inria. She started her career at Tractable building A products and the insurance industry at Schneider Electric. She has spent over 2 years in the AI hub driving the adoption of generative AI and developing solutions for asset management and electrical design. Now she’s part of the strategy and innovation team. She focuses on identifying and testing new AI technologies to shape Schneider’s future offerings. Passionate about AI, Julia is committed to exploring its real world business impact. I’m very excited to have you today, Julia.
Julia Peyre: Hi, Hello, Gosia. Thank you for having me today.
Motivation to join Schneider
Gosia: So Julia, we have founded the AI Hub inside Schneider. So the organization that is focused on implementing AI at scale at the end of 2021. You have joined two years ago. I was wondering what motivated you to join this organization?
Julia: Thank you Gosia, for the question. So to be honest, at the time I joined, it was in fact more a question of circumstances. So I moved to Grenoble and I was looking for a new job in industry to solve real world problems because that’s what I like to do. And as you said, it was about the times that the AI Hub was created to support business and function with AI and Schneider Electric was hiring people like me. So it just looked very much aligned. And I joined at this time as a data scientist. And now it has been in fact 2 years. I really like it. And as I get to know more and more the company, it’s actually becoming more and more interesting. And I do believe today that Schneider Electric has a key role to play in the energy transition party thanks to the electrification. So I’m very, very happy to participate in this.
Current role
Gosia: Now that’s that sounds interesting really. And what is your current role now in the strategy and innovation team?
Julia: Yes. So today indeed, I’m working in the strategy innovation team in the area. And our team looks in fact, in the future, our team tries to anticipate AI trends and what it means in terms of opportunity and risk for business. So I’m part of the strategy team, but also working very closely with the innovation team. And my role is first to contribute to the identification of strategy topics we should address with AI. And this is across all business units and functions. Then I look at the external ecosystem to identify innovative solutions we could use with a special focus on startups. And in the last part, when these two first steps are done, I work with innovation teams to test the ideas in practice together with the partners.
Look at innovation
Gosia: OK, I see among the people that I asked about the future of AI, a lot of them don’t like to reply to this kind of question because they say it’s so difficult to predict anything. There are new technologies appearing almost every week. There is some announcement. Could you please explain how do you look at innovation? Are you using any crystal ball? How does it work for you?
Julia: Yeah, so I have a practical way in fact to look at innovation. So if we take a bit of perspective, what is in fact innovation? What does it mean? So there’s two ways to look at it. Innovation is first finding new ways to solve a business problem, or you can also see it as inventing a new usage of a certain tool in the context of our business. So you see it’s about the tools that exist there to solve a business problem. The way I approach innovation is looking at these core ingredients starting by the problem. So what is the problem you address for your organization? It should have a high business value, it should be strategic for your organization, and it should also be well defined and formulated. And this is done concretely by talking to business units, data scientists, engineers, and clients to gather multiple perspectives so that you really understand the scope and the challenges precisely. And once this is done, you look at the tools, the solutions out there that you could use to solve this problem. And it’s where I recommend in fact, to almost completely forget about the problem, an open mind, not reject tools that might not exactly fit today because innovation is about something that does not exist today to solve the problem. So you should be open-minded. We have a team of creative people that are used to having these mindsets. And the last component is once we have this problem, the tools you have to put them together and test whether it’s working or not. So here it’s more about project management with a high uncertainty project. So it’s both about giving flexibility to your team so that they have time to iterate. But on the other hand, you don’t want to spend 12 months working on something impossible. So you have to also be clear on the definition of done and you need to know when it’s time to move on and maybe re-explore later.
Gosia: So in a sense, it’s better to fail fast and move to another solution, to another solution that you tell.
Julia: Yeah, I would say so even if you have to give yourself the time to innovate. So it’s a bit paradoxical.
AI Solutions: Make vs Buy
Gosia: Yeah. And I know that in your role, you also evaluate existing technologies. So what would you say is the proper balance between creating our own AI solutions in the company versus buying those solutions that already exist in the market?
Julia: So I really like this question because my opinion on this, it changed a lot recently. If you asked me this one or two years ago, I would have said just build the tool, because with open source tools, you can just build the solution yourself. It was obviously because I’m from a data sciences background. Today, I still think that open source is a great asset, but the make versus buy decision matrix is a bit more complex. And the way I like to look at it is in fact through an analogy. So allow me to do this analogy with you. So let’s say you have bought a piece of land and you want to build a house. OK, so the question is, what do you make yourself versus what do you make someone else do? So Gosia, small exercise for you. What would you do yourself on this piece of land to build your house?
Gosia: Oh, my God, I would select the plan of the house, but I would construct it with a company that does it professionally. And then I would probably decorate it myself. I wouldn’t like to use a decoration company to do this.
Julia: Yeah, I love your answer. So it’s true that there are some elements you want to buy to make built by other people. So for example, the foundation of your house, you want something solid, reliable that you are confident you can build on. So and it’s the same for our AI platform in the AI Hub, it should be reliable. So it’s a buy and we are indeed buying it from a cloud service provider. Now maybe there are some elements like the electricity of your house, for example. It’s a question of security. So if it’s done wrong, it can really be lethal. Personally, I’m not an electricity expert. I’m not certified for this. And even if I want to sell my house later, I need to have some kind of certificate of conformity so that new customers can trust the system. And in the hub, it’s the same. You can think of electricity as the data and the wires that transport this electricity as data pipelines which can be subject to cybersecurity attacks. So if this occurs, you lose clients. So again, it’s a buy. I would say in this case you have to go with partners who have expertise, track records, and can provide strong guarantees. We are using partners like Databricks, for example, on the data layer to do this kind of thing.
And now coming to the decoration part, you said you want to do it yourself. That’s an interesting question. You want to do it yourself because maybe you have some tools that can help you do this. So it’s feasible. It’s hard but feasible for you to do it. There’s not much security involved. Personally, I would do it. I recently bought a steam machine to remove the wallpapers and started the decoration. So with the right tools and learning from YouTube tutorials, for example, you could do it. But we can also say that removing wallpapers is not so fun. So you can also decide, for reasons of cost or time, and because it’s just not fun, to outsource it. But on certain things like if you want to decorate your child’s room with stars on the ceiling, now maybe it’s where in fact there’s some customization involved. It’s a creative task. You directly please your child. So now it might start to be a make because you want this specific control on where you put the stars. You also want to know the recipe that you used to maybe change the star location in the future. And it’s quite a lot of fun. So again, here you can see that when you have this more added value, this creative task, it can be a make.
So to conclude on this part, there are multiple factors involved. It’s about expertise, it’s about what your technical team can do. It’s about the cost, the time, the risks that you take, how much customization you want, how much added value you can bring yourself, and also how you envision the future development of your products. And today in the hub, we make this decision use case by use case and it’s important to remind these different factors.
Proactive review vs Presented offers
Gosia: Yes, indeed. In the end, it’s really a matter of making good choices and evaluating if I have enough expertise, especially the field and domain expertise. Do I have enough of the technical background to build something myself versus can I work with a trusted partner and use some of the solutions that already exist? So it’s always a decision-making process.
Gosia: And I was wondering, Julia, because in today’s landscape in most countries we have a very vibrant startup landscape. And I know that they reach out to us, presenting their new technologies and innovations. I was wondering in your job, is it more common that you are making a proactive review in which you are looking for some very specific solution and you want to see if it already exists on the market or is it more often that there are so many startups and companies out there presenting their solutions to us?
Julia: Yeah. So it’s a good question and we can come back to this definition of innovation that we gave earlier. So either you start from the problem and go to the solution, which is what we can call the top-down approach, or you start from the solution and imagine new usages. To be honest, today we base our planning on the top-down approach. So we’ll decide, for example, that each year we will address, I don’t know, four different problems that we identified as most strategic and critical to us. And each quarter we can start an exploration on one of these problems. This quarter, for example, we’re interested in tackling the challenge of lack of data for quality control. So we are looking proactively at different tools and partners in the ecosystem to address this challenge. We look at large vision models, synthetic image generation tools, advanced anomaly detection techniques. We contact different partners for this and decide to do a test with a few of them, compare the solutions so that we’re able to advise which technique we should use and also recommend partners. You see, that’s the first approach. But we might also miss interesting tools in the market that could help us unlock new opportunities because the field is moving very fast. Schneider Electric is also a big company with lots of different products and it’s hard to keep up with everything. So we also do bottom-up. We need to stay very open to new tools, welcoming new solutions, even if it’s not in our prioritized list. If an AI startup relevant to our business contacts us and pitches a solution that can help us solve one of our problems, we are happy to explore it.
Large corporations vs Start-ups
Gosia: And what are the different types of cooperation? What happens next? Is it more common that you work with large corporations or with startups?
Julia: This is a good question. So I would say think of cooperation as a relationship and you make your relationship what you want it to be, right? The first question you should ask yourself is what do I want? What is my purpose with the cooperation? Is it that I want to buy a tool? In this case, it might just be about buying a license. Or do you want to learn and gain expertise? In this case, it would be a cooperation where you have regular conversations with the technical team, for example. Or do you want to quickly test an idea? Or do you want, trying to understand, if you enter into cooperation with this company, what would it really bring to you with respect to your objective? Is it, for example, that you have the problem, you have the data, and they have the expertise to solve this? Or maybe it can be they have the ideas and you have the data. Is it kind of helping you? So with all these questions, what do you want with this company? You can first confirm whether you’re looking at the right partner or not and also understand how you want to cooperate with them.
So now I’ll give you a few examples in the AI Hub of different companies we are working with. We are working with big companies, as you said, more for technological partnerships. So basically we buy tools that are pre-built and that we can customize easily. So it’s a kind of self-service we support. For example, we would partner with Amazon to use Bedrock or Microsoft to use their Azure platform, open as a platform. That’s the first way we can collaborate with big technology companies. But technology companies today also have the best innovation teams. They have a pool of highly skilled engineers that are extremely efficient at the forefront of the latest technology and they are able to engage with us to solve hard business projects.
And so it’s called innovation partnership and we work together also to co-develop a solution. That’s actually what we did last year with Microsoft to build a solution for code generation for our automation engineers. So you see, even with big companies, there are different kinds of partnerships. Now we also work with smaller companies, startups, because a large part of innovation today occurs in startups.
Gosia: Yeah, exactly.
Julia: Yes. And so here you might have heard about our collaboration with Mistral. We want to understand how we can use their model in our different use cases, in particular to improve efficiency. We were actually part of the early testers of Mistral Large earlier this year and are continuing the conversation. So you see there’s quite a lot of possibilities. But with big tech startups, you can also think of consulting as another way to cooperate. If you want to quickly test an idea and you don’t have the technical resources, for example, you can also think of academic partnerships. We are also doing this in our team, so there’s a wide range of cooperation. But what is important is to remind the two questions: what do you want and what is the partner in front of you offering?
Gosia: Yeah, I think it’s a quite important message because in the past episodes of our podcast, we were inviting people from different businesses and also the different internal functions of Schneider and they were describing really a variety of AI solutions that we develop inside the company. But what you’re saying is equally important is that this is such a vibrant and moving space that you have to have this relationship, you have to be aware of what’s happening on the market and there are some solutions that we really appreciate building on and customizing ourselves. But you need to have these technology partners around you.
Julia: Exactly.
How to test new AI technology
Gosia: And the next phase then, once you have selected a potential partner, is to evaluate a solution. And I would be really curious to know, how do you evaluate, how do you test new AI technologies?
Julia: Broadly speaking, to test new AI technology, you can consider three main criteria. And you really test these criteria all along the process, right? So first it’s about the team, then it’s about the technology, the solution, and finally, it’s all the question of integration.
But the team, the company, you want to understand whether this company is serious, trustworthy, so who they are, how many are there? Do they have some track records? How long have they been around? It’s also about how you feel about them. What are their values? Are their values aligned with our values? Do they communicate transparently? Because communication is most important, good communication, and then a bit of financial. So are they backed by trusted investors? Do they have enough money for the next few months, years hopefully? Or do they even make their own money today? And this is important, this financial question, because you want to make sure the startup won’t be out of business tomorrow. You want a long-term relationship that is supported by this. It actually reminds me of an important question because my first job in a startup after my PhD lasted only three weeks. Yeah, the startup ran out of money. And you know, when I was out of my PhD, I just didn’t ask enough questions about the financials. I was just excited by the project vision. So I guess that’s the lesson. We don’t want this to happen. We are happy also to consider early-stage startups, but we need a bit of visibility to work together. So that’s what I did in another startup just after this one. But yeah, Schneider Electric is more stable, let’s say.
So that’s about the company. And after this, it’s about the technological solution. From a technical point of view, it usually starts even when the startup does a demo to you. You can also ask for more technical documents, papers, patents, you can look at these. But the core of the test will be done through a proof of concept on one of our use cases. It should be on one of our use cases because you need to see if the real solution can work on toy data and not work at all in a realistic setup. So here what I would advise is really being mindful of picking the right use case and metrics. It’s about being clear on what you want to test, especially how you will compare the solution to. Like basically, what is your baseline? Because if you don’t do this preparation well enough, what will happen is that the proof of concept will just work. It will give success, but it’s not necessarily because the solution is good, it’s because the evaluation is weak. It’s not good for you, it’s not good for the startup because if it fails in production, you have to redo everything again. So be really rigorous in this proof of concept, the metrics, the baseline. And this is something when you’re a bit new to AI, you don’t realize how important it is.
So we’ve talked about the company, the technology, and the last part is about the integration. How will the solution be integrated into your workflow after the technical proof of concept is validated? Will it scale well? How much will it cost? Will it comply with your data and cybersecurity policies? So all of these questions for innovation partnerships, we look at them, but it’s not the core of the test we’re doing, but we have to look at them as well because the end goal is of course to integrate the solution into our product at scale.
How to make relationship successful
Gosia: Yeah, I think that’s great advice. And I’m looking at the clock and actually we are running out of time. But I still wanted to ask you one last question. What do you think is important to make these partnerships and collaborations successful?
Julia: There are a few elements that we already discussed here. So for me, a successful partnership is one where the relationship lasts. And together we have built, we have solved the business problem that we integrate into our products at scale. So for the relationship aspect, it’s really important to be clear about the objective of the collaboration. You’re always thinking of a win-win for each party. Your partner’s success is your success in an innovation partnership. I think that’s especially important because maybe the partner won’t have a solution for you today, but they might have tomorrow. So it’s important to build this relationship. That’s for the relationship. And now for more like the success of integrating your solution into your project at scale, what I found important is to work ahead with the business units, all the engineering teams, the data risk team, the procurement team, all these different teams that will help the integration to succeed. And that’s especially important if, like me, you are in an innovation team that is a bit transversal, not incorporated into a business unit.
Wrap up
Gosia: I see and I really like what you said about our success will be our partner’s success and I think we can finish with this for today. Thank you so much for your time. I appreciated all the insights and I hope that our audience also found them very insightful. Thank you, Julia.
Julia: Thank you Gosia for having me here. It was a pleasure.
Like what you hear?
- Visit our AI at Scale website to discover how do we transform energy management and industrial automation with artificial intelligence technologies to create more sustainable solutions.
- Listen to the other episodes of the AI at Scale podcast.
AI at Scale Schneider Electric podcast series continues!
The first Schneider Electric podcast dedicated only to artificial intelligence is available on all streaming platforms. The AI at Scale podcast invites AI practitioners and AI experts to share their experiences, insights, and AI success stories. Through casual conversations, the show provides answers to questions such as: How do I implement AI successfully and sustainably? How do I make a real impact with AI? The AI at Scale podcast features real AI solutions and innovations and offers a sneak peek into the future.
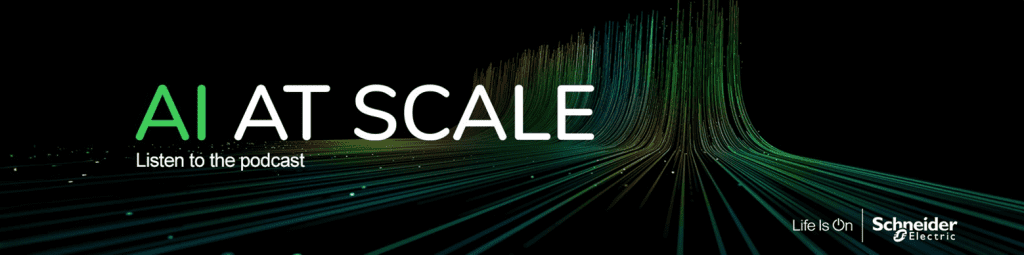
Conversation
As a student,i’m very amazed by how well your organisation use effectively AI to transform the industry field and the sustenability.