This audio was created using Microsoft Azure Speech Services
Let’s talk AI ethics
What does it mean for AI to be ethical? Ethics can be perceived very broadly. In the AI world, it can be associated with different aspects such as trustworthiness, responsibility, cybersecurity, algorithmic justice, etc. In this episode of the AI at Scale podcast we welcome Mia Shah-Dand, founder of Women in AI Ethics, and CEO of Lighthouse3 to define key aspects of AI ethics and find practical approaches to achieve it.
In the first part of the conversation Mia explains that AI should be treated as any other customer facing product and comply with universally accepted set of rules. She mentions laws, regulations, and ethical code of conduct which support finding the right approach, protecting users from harm and risks.
One of important aspects of AI ethics is to include women and other marginalized groups that are traditionally underrepresented in AI. In the second half of the show, we highlight what women bring to the AI space even though they are still outnumbered according to the recent statistics. Being a founder of Women in AI Ethics, Mia shares different initiatives such as the 100 Brilliant Women in AI Ethics™.
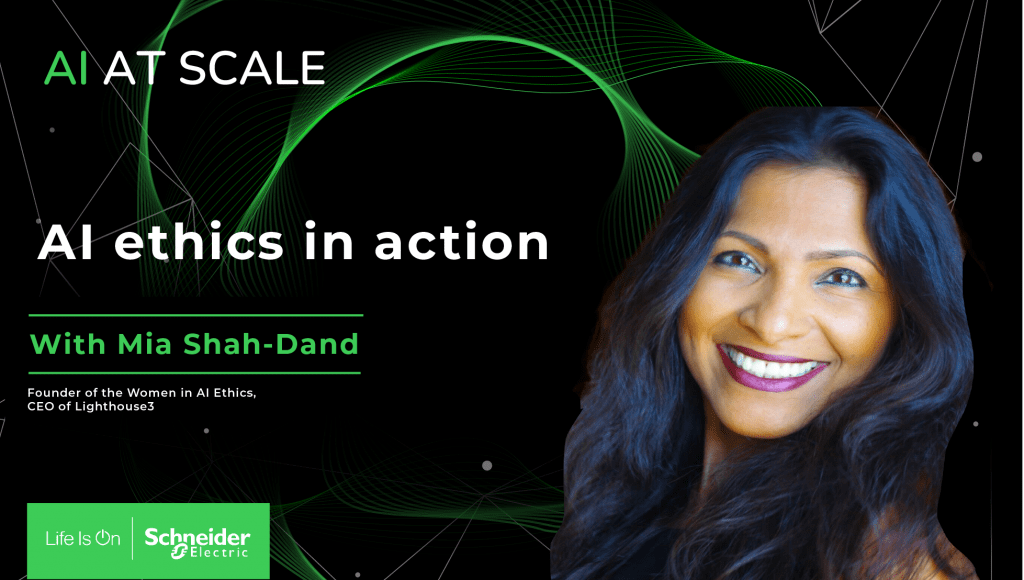
Listen to the AI at Scale podcast
Listen to Mia Shah-Dand: AI ethics in action episode. Subscribe to the show on the preferred streaming platform (Spotify, Apple Podcasts).
Gosia Górska: Welcome to our AI at Scale podcast. My name is Gosia Górska, and I was looking forward to discussing the topic of AI and ethics for some time already. I am very pleased to introduce my guest, Mia Shah-Dand, the founder of Women in AI Ethics, a platform promoting diversity and ethics in the technology sector. Mia is also the CEO of Lighthouse3, a technology advisory firm based in New York and California. She has an exceptional track record of leading multidisciplinary teams and advising large enterprises on the responsible adoption of AI and new technologies. She serves on the AI Ethics and Society Conference program committee for 2024 and the advisory board for Carnegie Council’s Artificial Intelligence and Equality Initiative. Mia is really known for her deep commitment to advocacy for a more ethical and inclusive tech future. Her work has been featured in Forbes, Politico, Fast Company, and BBC. Welcome, Mia. It’s a pleasure to host you today.
Mia Shah-Dand: Thank you, Gosia. It’s great to be here.
Gosia: Now, Mia, I think we need to start with a definition, because AI ethics can mean so many things to so many people. What kind of aspects would you include in the definition of ethics in AI?
Mia: You’re right, Gosia, it does get very confusing because there are so many different terms being used out there, and there’s AI ethics, AI responsibility, trustworthy AI, and so on, right? So it can get a little bit confusing. So let me start with AI ethics. So let me start with a question: Are you an ethical person?
Gosia: I like to think so, yes.
Mia: You ask anyone that question and their response is typically yes, or ‘I try to be an ethical person,’ because it’s so intrinsic to us as human beings to be ethical, but we all also define what that means for us differently, right? I am vegetarian. My friends are vegan, and some eat meat, some eat only fish, and so on. So we all define what that means and where we draw the line. But as a society, as a nation, or even transnationally, we have come up with some standard definitions of what that means. What does ethics mean in terms of human rights, civil rights, how do you protect children, and so on. So we have come up with ways that we can protect others by being an ethical society. So that’s what we all want, individually as well as collectively.
So in an organization, when we talk about AI ethics—first of all, it’s a very broad discipline. So AI ethics includes—the way we have framed it—is how does AI impact civil rights? How is it designed ethically in terms of civil rights? So we look at AI safety and making sure that we consider harms, environmental impact. So we are looking at a very broad definition of AI within the framework of all of the different dimensions that AI can cause harm or introduce risks.
However, within an organization, organizations look at AI ethics and should look at AI ethics in the framework of not just ‘this is nice to have’ and going above and beyond, but mainly around what does the regulation say? What does the law say? How does that apply to these technologies? But in the absence of those, companies are deciding their own responsible AI policy and trying to cover that gap by looking at their internal, say, standard of conduct, say, ethical policy. I’m assuming Schneider also has its own policies.
Gosia: Yes, of course. Of course we have. And we even created a dedicated set of rules for AI with a dedicated AI responsible committee board.
Mia: Amazing. See, that is the right approach, that you have to create a framework which is also grounded in what is universally accepted and agreed upon, because companies cannot be just making up their own rules. So that’s number one. We have collectively come up with certain laws and regulations and ethical codes of conduct, and organizations have then adopted those within their own context. So there might be some differences between how, say, one company approaches this versus another. But universally, we are pretty much on the same page as to how they should be deployed.
The challenge that comes up with AI technologies, because of the hype, they’re treated as something special. They’re treated as, ‘Oh, this is this magical thing, so different rules apply to it.’ And I would push back against that. What AI ethics and the broadest scope of responsible AI is really does not change depending on whether or not this technology is AI or any other flavor of AI, because AI, again, is a very broad field; it is not just one thing. So I would say the way companies should be looking at AI is pretty much in the context of the broader scope, which is much bigger than at a level of national and humanity, but also within the organizations, how they can be applying these same principles to protect their customers, protect the users, protect the organization from harms and risks of AI.
Practical approaches to ethical AI in business
Gosia: In terms of practical approach, you mentioned that, of course, many businesses, they don’t do any harm on purpose. Yet AI is still such a new technology that companies are implementing. And in many cases where we heard about some unintended harm that was happening, it was exactly because the company wasn’t thinking about what are the potential risks and how the practical AI application will be used by the customers. So what are the practical areas, how companies can actually think about ethical AI? Ethical approaches like, for example, would you say that cybersecurity or model monitoring against a drift is a part of ethical approach that companies should have?
Mia: Great question, and it’s multifold. So I’ll talk about the different parts that you have to this question. So let’s start with how do you apply these principles in practice, and why companies struggle with this—and it is a challenge—is that AI technologies are presented as these black boxes. There’s a lot of misinformation. Some of it is intentional, some of it is just ignorance—that people don’t know what AI even is. AI is also a very broad field. If we think about right now, the popular narrative is AI is just generative AI, and everybody is talking about just generative AI. But there’s a lot more to AI than just generative AI. But we’ll stick to just one part, right, because we only have 20 minutes.
So if we look at artificial intelligence or just look at generative AI, we have to start looking at how are these models constructed, right from the inputs that go into these systems to how these are processed and what parameters are used to develop them, and then how these are deployed in what context, because you can have harms at every stage of the process. However, when organizations adopt these technologies, they are literally just sold as, ‘Oh, here’s the magical box. It can improve productivity. Here are the amazing things it can do,’ and without any explanation about where are you getting the content for your generative AI model? How are you making sure that this content is—you have consent to use this content? How do you make sure it is not private data? How do you make sure that this content is not biased? How do you make sure this content, when we deploy the system, that it is not going to harm our people? It’s not going to create risks, right?
So the lack of knowledge about these technologies leads to ignorance about how these harms should be managed. And there isn’t any excuse for this, very simply, because while the tech vendors, right, they have a vested interest, they’re not going to tell you what’s in there. No one is going to sell you a product and say, ‘You know what? Here are all the harms of these products.’ They’re not going to tell you that. But the good news is there are a lot of women in this field, AI ethics field. When I started this work back in 2018, they were sounding the alarm on the—these technologies come with harms, right? So right back then.
So we fast forward to 2024. When you start applying these AI ethics principles, I always ask organizations to start with your existing policies. You have data privacy, you have data compliance. And if you’re a large organization, there is no excuse for not having those policies already in place. Then you start looking at—you already, right? You already have a foundation, because again, if you don’t already have a data privacy or you don’t have a compliance team, that’s a different question. You know, that’s a different conversation we should be having right now, because that’s a bigger problem. So then if that’s being an AI problem, you have an organizational problem.
But going back into AI, for any large organization, you should already have your basic infrastructure to protect your customers, protect your organization, protect your employees. You should already have those safeguards in place. There is no excuse for not having that. And then you start looking at, ‘Okay, I already have these in place. What framework, existing frameworks can I use to manage these new technologies that are coming into our organization?’ And then look at where are the gaps? Where should we address those gaps? So what questions don’t we know? What are the answers we seek? And then this becomes a much more meaningful conversation.
And all this, I’m a big fan of inviting the legal folks into these conversations right up front because they’re always invited when, oh, things go bad. Ethical principles into practice—it has to be a team effort. It has to have representation from risk, legal, compliance teams. If you have a recruiting software that you are basically only—you’re deploying, if it’s going to affect your employees, you can’t talk about productivity without including your HR teams. You cannot have a hiring or recruiting platform or system without including your recruiting team. You also have to think about what are the implications of these technologies on your IT infrastructure, your existing infrastructure. What are the risks? Employee training is a big part of it. So those are all the big pieces that we make sure that are in place when we apply these technologies.
And then last but not least, you asked about, okay, what is model drift versus cybersecurity? So the framing of these is a little bit different, because ethics is about harms and risks to your customers, your end customers, your internal customers and stakeholders, your employees. Here’s the funny thing. I was reading about how these tech vendors, these big companies, were selling these AI products. They were having to warn their employees about not sharing proprietary information into generative AI models. They said, ‘Stop putting sensitive information in there,’ because what they realized is they’re selling these products to everybody and claiming magic, but their own employees were putting all sorts of sensitive data and information into these systems, and these systems are not secure. They can be hacked. That information can get into the hands of your competitors. You have to be very careful as to what you’re putting in there. And even the tech vendors don’t realize that, and they are not thinking about those, which is a bigger problem.
And then when we talk about harms, that is an ethical issue. But protecting your organization is literally a business issue. Like, it’s a big critical issue, because when your employees don’t know how to use these technologies or they don’t know how to secure these technologies, it puts your entire organization at risk. So somewhere we go from ethics becomes a big business problem if it’s not taken seriously by the organization.
The role of women in AI ethics
Gosia: Yeah, that’s right. And I’m happy that we seem to be completely aligned on those matters. And I really like to hear our AI leaders at Schneider Electric when they say, at some point, AI is not different from any other products. We should not forget about basic compliance, basic risk management. This is like, you should treat AI technology as any other product. You should prepare for the risks. You should evaluate. You should have policies in place. And people tend, as you said, to treat sometimes AI as magic. So they don’t think about the risks. They just expect some miracle outcomes out of using it. So definitely, I think the practical advice that we can give to everyone is treat AI technology just like any other product, especially software product. You need to be aware of the risks, you need to manage the risk, and you need to be prepared for this.
And following up on this, I was wondering when you started Women in AI Ethics initiative, and you mentioned a bit already about the fact that you were hearing—you saw at some point some of the signals coming from the women researchers about the risks in terms of inclusion, in terms of other risks coming from AI. Why you started this initiative and why actually you thought about directing it or concentrating it on women in AI? Like, are women bringing more value or simply a different value to the AI world?
Mia: Another great question, and I’m so glad to hear we are aligned on so many of these critical questions we face when we adopt these newer technologies. And when I started my career—and I worked in Silicon Valley for many years, so I live in New York right now—but I started my career when digital technologies were being adopted and companies were really trying to figure out how do we manage our digital transformation? How do we adopt these new technologies and do it responsibly? Because again, it’s the same—we follow the same cycle every time. There are new technologies that come into the space, and organizations are trying to decide how to best deploy them for business.
So my career started there. So I’ve had experience both on technology side, like adoption of technology, but also adoption, setting up governance models, user training for those new technologies. So when I—and I’ve worked for many tech vendors as well, like in the tech industry. I used to blog; I was a tech blogger for many years. So I’ve taken an inside look, a very close look at a lot of these tech companies as well, just part of my work, both on the corporate side and also in media.
So what you notice were a couple of things. One is, like you said, AI is like any other product. No matter how much they dress it up as this magical thing, right? Any products out there that I know of that an organization would just take in-house and adopt without safeguards, right? And not even technology. Think about it this way. Let me give you an example. Do you drive, Gosia? Do you have a car?
Gosia: Yes.
Mia: Okay. Would you buy a car without a seat belt? Or does your car even have, like, airbags without airbags?
Gosia: No, I don’t think so.
Mia: No. Okay. So you wouldn’t want a car with air—right? You wouldn’t buy a car—I mean, who in their right mind would do that? And yet, tech vendors believe that they can just throw out these products, really flawed, biased, which they call it hallucination, these AI products and generative AI hallucinates. No, it’s just not accurate, and it’s biased, and it’s making up these—it’s just making up stuff. We would have never accepted that. There was a time when tech vendors would introduce products and it would have, like, alpha, beta, and say, ‘Okay, there are some flaws, but we’ll let you test it.’ Now, it’s just lack of respect for the end users, lack of respect for people who are going to be harmed. They just throw out these products out there and say, ‘Okay, you figure it out. We know it’s flawed, but it’s your problem. It’s magic. It’s a black box.’ We would have never accepted that as an organization, right? Like, if you think about, like, ten years back.
Gosia: Yes. And as a consequence, we see some of the companies actually removing the product from the market because of the comments coming from the customers.
Mia: Right, exactly. If I were just to say, these are terrible products, and not just from an ethical perspective, from a business perspective, they’re just terrible. They don’t have that level of accuracy that the tech vendors say they have. They still—I do a lot of testing of these products because I have to, before we can recommend these products to any client or customer, we have to test these.
So that was a big issue. What I noticed was two things working in the tech industry, that this narrative: there are no women. There are no women. Again, statistics—I’ll share some facts—33%, like one-third of the tech workforce is women. So there are women. They might not be in the majority dominant position like men, but there are a lot of women. And then I noticed in around 2018 when Dr. Joy Buolamwini, Dr. Timnit Gebru came out with their paper Gender Shades, which showed that facial recognition technology, some of the most popular commercial systems, were not accurate for Black women. They had lesser accuracy for Black women than they did for white men.
Now, think about it this way. So you have a product that is designed only accurately for white men. It would be okay if you just lived in a world which is full of just white men, and that’s it. That’s your only audience. But who only sells products to only white men? I mean, this is a product that’s completely flawed. So even from an ethics perspective, it’s wrong. It’s just not okay. But even from a business perspective, why would you buy a flawed product? Why would you buy a product that’s not accurate for your entire customer base? It makes no sense, right? It is not logical, it’s not practical. It is irrational in a lot of ways.
But the reason that narrative still persists—’there are no women,’ that women are not qualified and all of that—and yet I saw in 2018 that women are leading the way in pointing out these technologies are flawed, they are unethical, they are biased. There are so many issues with them. So that’s where I published a list, which is 100 Brilliant Women in AI Ethics list, to raise awareness that, hello, there are women, hundreds and thousands of women, who are not getting the visibility about the issues they’re raising. Fast forward to 2024. Here we are. Everyone wants to be an AI ethicist. Everybody’s talking about AI ethics right now. It’s become more common and more acceptable to have those conversations. But let’s not forget the women who did that work, who got us there. So this is how my corporate—my work as a tech advisor, also my work as an advocate for diversity in tech, and my tech blogging as media—all came together and converged at this space where I am a tech consultancy, which is Lighthouse3. We are based in New York and California, and also Women in AI Ethics, which is a global program that I’ve started back in 2018.
Gosia: I’m so glad, Mia, you mentioned this, because I was actually watching a video when the researcher, Dr. Joy Buolamwini, she was testing a face recognition application. So the robot was supposed to recognize a face of a human, and she was putting a white mask on her face, and then the robot was able to recognize her as a human. When she was taking off the mask—and obviously, she is of dark skin—the robot was not able to recognize her as a human. And I realized how much harm this application can cause and how ridiculous it is that you put on the market something that is not able to recognize a big portion of society as humans.
Actually, let’s go to the second part of our conversation, which is more about AI ethics in practice. So, as you mentioned, you launched a startup that is focused on responsible AI. So what kind of gap you saw on the market, and what kind of services do you think customers need in this space?
AI ethics in practice and responsible AI adoption
Mia: Amazing question, because I think about this a lot, because where we see is making sure that we always look beyond the hype. So that is my background, right? Working in that price space—you and I, Gosia, are the same, right? We think similarly because when we work on products, whether we are adopting products, whether we are using products that impact with large organizations that touches hundreds, thousands, and millions of customers, we are not working just one-on-one.
In my tech consulting role at my firm, what we look at is the same as we do with any emerging technology, because we didn’t just start this work yesterday. We have been doing this for well over a decade because there are so many people who are now suddenly in the AI space—they just started like last year or two years back, they were in some other crypto space or some other completely unrelated field, and they just decided that we are going to work on this because there’s a lot of hype. We’ve been at this for a long, long time. My co-founder, my partner actually specialized in artificial intelligence 30 years back. So we are deeply steeped in a lot of these technologies that we talk about.
And we start with always the number one question is, does this technology help your business goals? Will it help you achieve your business goals? Right. That’s number one. We always start with the very basics, because if it doesn’t and you’re just adopting a technology because it’s cool, or you met some tech vendor and they said it’s amazing, that is not going to help your business. That’s number one. So then we look at, is the product delivering or the solution delivering what the tech vendor says it’s going to deliver? And there’s a lot of opportunity and challenges and opportunity in that space.
That’s the gap I see—the lack of transparency, the lack of benchmarks, the lack of any information about whether or not these technologies are delivering is such a huge issue right now for a lot of companies. And that’s where we focus our effort, because we are seeing report upon report and hearing from our own customers and clients that we work with, is that they are abandoning a lot of the AI projects because they are not seeing a return on investment. So the tech vendor sells you on a product because that’s their job; that’s what they’re trying to do. The hype—definitely they’re fueling the hype that their technology can do this, AI could do that. But when it comes to adoption of these technologies within the organization, that’s the reality check. That’s where we help customers decide, did this product or this solution work as it’s supposed to? What kind of benchmarks will we use in order to make sure that this does meet our internal benchmarks? What do those benchmarks even look like? What should we be—is 80% accuracy good? Is it 85%? Should it be 90%? Because they said—the vendor said 90%, but this only has 75% accuracy. That is not acceptable, right?
Some of those basic things, like even doing the sanity check and reality check of these—vetting these products is important, and then running the pilots, making sure that those—if the pilot worked and it’s successful, then how do you scale it? We take it to the next level. But the big piece of the work that I personally get excited about is how do we bring together these diverse stakeholders, all these different functional groups, and make them smarter about AI, right? From procurement to—right—to your finance, risk, legal—like, everybody in your organization needs to be so smart about these technologies that they can ask the right questions, that they are looking at these technologies and seeing, ‘Okay, does this work for my business? How will I deploy it?’ And we also help with user training. And again, that’s the part I’m passionate about, whether it’s analysts—I did a training for HR analysts, people—actually, the HR team and all the analysts that support it—and I walked them through how biases get into AI systems, how you can find them. And they did not even think there were so many things when you’re even reviewing or recruiting, like a resume, that they don’t think about like, ‘Oh, this is how the AI systems can be biased against people who live in this zone, even zip code,’ right?
So there is so much more to AI technologies when it comes to adoption in the enterprise that these organizations need help with. And that’s where I feel like is the opportunity, and that’s where we help them.
Best practices for implementing AI ethics
Gosia: Yeah, and it’s really great to see the first companies that are filling this gap, but also some practical tools that are proposed by different organizations. I recently came across an evaluation tool that is proposed by the European Commission. It’s called Assessment List for Trustworthy Artificial Intelligence, because I’m studying AI also at one of the universities in Poland, and we were actually practicing this. We were imagining an AI application, and we entered the tool that is available online. And by answering certain questions, we got to the point where we got a visualization of the ethical assessment of our application.
And what was really clever about it is that, of course, it’s a bit—you know, you need to put a bit of work and thinking to reply to all these questions. But at the end, you get a clear image of what is the area where your application can cause some risks, what are the areas that you need to take care of in order to have your application be ready on the market to meet customers and not cause any unintended harm, for example. And it can be very simple. They are asking questions about privacy, data governance, transparency, but also accountability. Do you have a process in place if something goes wrong when the customer is using your application? So these can be very basic things, and I hope that this kind of tools will be appearing and that everyone who is working on AI but also using AI will be more aware about these risks and what can we do about them.
Mia: Absolutely.
Gosia: So my last question, maybe, Mia, would be, what are the best practices to implement AI ethics into business practice? You mentioned a little bit about those approaches and those steps, but where should companies start when they start to apply AI?
Mia: First of all, I have to say the tools that you mentioned are very helpful and valuable, and simply because they nudge you to be more thoughtful about these technologies. They force you to think through each decision and how these technologies might impact different stakeholders, how it impacts your business, and where those harms might exist. I feel like that is such a valuable tool. And again, I would love to see more of these tools available.
I feel where our work really starts—and I have to put this out—that AI ethics is not glamorous work. The work I do is not glamorous. I love what I do, and the reason for that is it is really about figuring out—you’re slowing people down, I’ll be honest. You’re slowing people down so that they can run faster, and I’ll tell you how that works. A lot of work that I do—the unglamorous work—is about protocols, processes, setting up the structures, and setting up the environment so that people can take—move faster. They can take more risk in having an environment where you could test things. I’m a big, big believer of testing things. But for that, you need a process where even the technologies that you’re testing have to be vetted. And once you have a good set of technologies, you’re like, ‘Okay, I have to solve this business problem. These are the technologies that are going to help me do that.’
Where the best practices I strongly, strongly encourage companies to do—you set up that space, it can be a lab, or I set up a center of excellence for global clients. It’s very important to have a space where you can not only—not just a research lab, but also a space where all of these ideas for how technologies can be used can come—can be curated, and then you can then deploy and scale them across all of your organization. For companies which have a global presence, have multiple divisions, and are large organizations, the key issue is always, how do I take this technology? How do I take these technologies? How do we then deploy it in a way that everybody in the company benefits? And how do I also take it to my customers so customers can also benefit from it? Like, I talk about digital transformation, I feel AI transformation, but responsibly—that’s what I look at.
So the best practice is really create that environment where you can quickly test these products, you have benchmarks, you know exactly what kind of thresholds you’re looking for, what kind of performance you need. Our work really then starts with—where my company really works on is—and where we are building the product—is at the performance management side, where performance management of all of these different models and comparing, are they delivering what we thought they should be delivering? If not, what changes do we need to make? What kind of updates do we need to make? What kind of revisions? How do we need to change things? But you can’t do that unless you have all of that set—the infrastructure, the environment, the organizational structure, and of course, support from leadership to do it in a very organized, thoughtful manner.
Because one thing I’ve heard from a lot of industry reports and also with interviews with business leaders is this emergence of shadow IT. And what that is, is organizations which are not related to IT are just taking these tools and deploying them in their own departments without any governance or protocols. And that is a little scary because you don’t know what these models are doing. You don’t know what kind of risks and harms they’re presenting. So I do believe in a very centralized, organized approach which can support a decentralized organization. So that essentially, I would just leave you with this—organized, centralized approach for a decentralized organization which allows you to move faster and scale AI more responsibly.
Final thoughts on AI ethics
Gosia: Yeah, and it’s a great way to name the fact that you need to slow down to do some of the not very glamorous things in order to then speed up and be able to use this technology at the maximum of its value. Interestingly enough, in one of our episodes where we invited Alison Sagraves, she was calling it that ‘boring is the new sexy,’ actually. So there is this job that needs to be done in the background so we can actually use safely this technology and really benefit from it. And I’m very happy to see the initiatives like the one that you manage about having a list of women in AI that you can invite to a conference, that you can ask for opinion, you can hire them, so they actually bring their expertise and their fresh approach to AI.
So let’s finish maybe with this. How do we bring more women to AI, Mia?
Mia: Oh, I love this question. What a great way to conclude our conversation. Women are already here, right? They are in HR, they are in marketing, they are in all sorts of functions. They are also in your technology teams—they’re fewer, but they are there. Therefore, we encourage organizations to meet women where they are at and deploy their expertise. We work in many different fields. Women have an expertise in functional expertise that makes technologies better.
And the reason women are more effective at building more responsible technologies is because they notice when things are not built for them. You notice—I was talking to someone who—we were talking about how if cars were designed for women, wouldn’t we drive more? Right? Like, it’s like that. When things are not designed for you, you notice. And that’s what the value women bring. When a technology is designed for them, they are more likely to see what the ethical blind spots are. But also, we have so much more diverse expertise. We are not just builders of technologies; we are designers. We are the ones keeping us safe. We also bring diverse functional expertise which can help these technologies avoid blind spots.
I believe the way to do it is we have to change our perception of who is an AI expert, and we do it by recognizing the diverse expertise of women without trying to force-fit them into this, ‘Oh, you have to be a computer engineer or you have to be a data scientist.’ There are these narrow roles that women are forced to fit into to be considered AI experts. It’s time we push back against that and say the expertise that women bring into the space itself is valuable, and it will—that diverse expertise is what’s going to help us—help us build more responsible, trustworthy, and inclusive AI systems.
Gosia: And I hope that the fact that actually AI is omnipresent and we are really currently building the future of this technology by working in it—this is something that would encourage more women to be interested in this field and also bring the competencies, the expertise that they have from different fields to AI. So this technology is actually created inclusively for a better future for everyone.
Mia: 100%. I couldn’t agree more.
Gosia: Thank you. Thank you, Mia, for spending this time with us. I would love to continue. I hope we will meet again, maybe in the podcast or at another opportunity. But thank you very much for your time today.
Mia: I look forward to it. Thank you Gosia for having me.
Like what you hear?
- Visit our AI at Scale website to discover how do we transform energy management and industrial automation with artificial intelligence technologies to create more sustainable solutions.
- Listen to the previous episodes of the AI at Scale podcast.
- Read more about Women in AI Ethics™.
- Discover the The Assessment List for Trustworthy Artificial Intelligence (ALTAI) by European AI Alliance.
- Check out two blogs about Women in AI mentorship program at Schneider Electric:
How having a mentor helped me in my personal development?
More women in AI are needed for an inclusive future we all desire
AI at Scale Schneider Electric podcast series continues!
The first Schneider Electric podcast dedicated only to artificial intelligence is available on all streaming platforms. The AI at Scale podcast invites AI practitioners and AI experts to share their experiences, insights, and AI success stories. Through casual conversations, the show provides answers to questions such as: How do I implement AI successfully and sustainably? How do I make a real impact with AI? The AI at Scale podcast features real AI solutions and innovations and offers a sneak peek into the future.
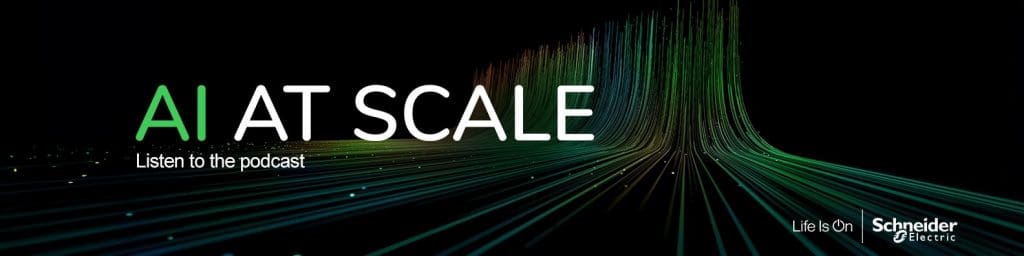
Add a comment