If someone asked me a year ago what are the biggest issues that companies struggle with when implementing AI, I would probably have highlighted data quality and availability. And while a growing focus on data and progressing digitization has helped organizations to start addressing these challenges, Gartner still project that around 85% of all machine learning (ML) projects will fail.
In this context, I see a bigger challenge confronting C-level executives responsible for driving innovation and efficiency. AI has become a “hype topic”; and while everyone talks about it, not everyone fully understands it. Put simply, to grasp the full potential of AI – and understand its inevitable limitations – you need to pilot multiple projects and fail enough times to draw meaningful conclusions.
But let’s face it: in harsh economic conditions, few companies have the resources required for such experimentation. At a recent AI event, I overheard: “We have more pilots than an airline, yet we are not an aviation company”. It underscores that many companies are motivated to use AI. However they fail to narrow down their use cases, achieve real impact and return on investment (ROI).
So how can this be addressed?
How to implement AI and achieve return on investment?

1. Looking for ready-to-use AI solutions
First, and most obviously, when a ready-to-use AI solution exists on the market, it strongly accelerates ROI. A good example is chatbot software.
Chatbots are AI-enabled programs that use natural language processing and machine learning algorithms to simulate human-like conversations with users. Companies can integrate chatbot software with its website or messaging platforms. It can save time and money for customer care service.
On the other hand, many of the ready-made AI solutions quite often entail some implementation burden. Why? Different businesses operate in different regions and industries. They usually specialize in different niches within a single industry. In that way, those companies face very particular challenges that only substantially customized AI solutions can begin to meaningfully address.
Put simply: when it comes to AI, one-size not always fits all. This is especially the case in industrial environments. There, AI solutions often require customization that takes into account a unique set of factors.
2. Tapping into tailored solutions with domain experts
For these reasons, tailored solutions can often be integrated more seamlessly into a company’s existing systems and processes, minimizing disruption. Therefore, to get the full value of AI and maximize ROI, developing a bespoke solution with a domain expert can be the best approach.
Imagine a consumer-packaged goods manufacturing line that you want to optimize to be more energy efficient. A customized AI algorithm suffused with high frequency production data can capture variables related to low energy consumption, high productivity, and automatically optimize machine settings. Machine learning model can monitor a prescribed individual production plan. It can detect anomalies and further optimize settings according to the plant’s particular requirements.
This kind of scenario underscores the importance of customization when implementing AI in complex industrial manufacturing and energy management environments. This is something we observe everyday with our customers. By attending to the particular needs of particular businesses, AI can then fulfil its capacity to increase efficiency, accelerate decarbonization and deliver ROI.
Driving business value and sustainable impact
In manufacturing, some of our clients aim to produce the most sustainable product in their range. Some focus on price, and others on the specific quality of their product. With AI we can analyze all variables to formulate an optimal manufacturing set up that can help to deliver the most sustainable, cost-effective and highest quality product possible.
How many industry players rely on manufacturing systems designed in an cheap energy era when carbon emissions were uninfluential? The technology needed to address these issues is available to us today.
And, as we’ve gone through optimization projects with many clients, we have observed a considerable margin of improvement that is ready to be tapped with AI and data analytics. Data interpretation and field expertise are key to identifying these opportunities.

Driving efficiency with AI solutions
Our domain expertise, combined with our deep knowledge of artificial intelligence, positions us strongly to develop AI-enabled solutions from conception till implementation.
We assist our customers with both off the shelf AI solutions and tailored, agnostic solutions, in order to unlock efficiency and ensure meaningful ROI on AI projects in the fields of electrification, automation, and decarbonization.
AI is here to stay and it can yield savings and lower carbon emission over months, years and decades following implementation. That, surely, is the kind of lasting, sustainable impact after which any forward-thinking executive should be striving.
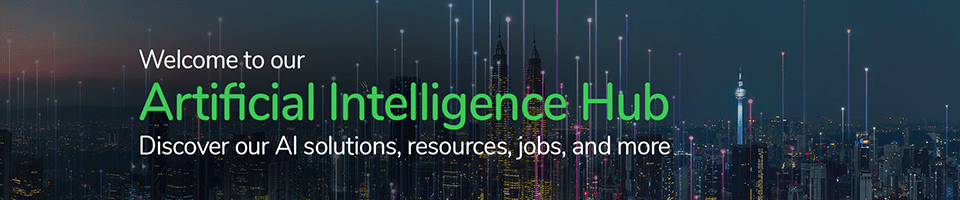
Want to know more about AI at Schneider Electric? Read more from our Chief AI Officer, Philippe Rambach and discover how we do it on se.com/ai.
Add a comment