This audio was created using Microsoft Azure Speech Services
AI is rapidly changing data center workloads from large training clusters to small edge-inference servers. The new AI landscape is driving up rack power densities, in turn presenting new challenges across data center infrastructure. This increased energy load is challenging all data center operators, especially colocation providers that serve large enterprises and hyperscalers, to look at new strategies and technological advancements to keep up with their sustainability goals and customer performance requirements.
At Schneider Electric, we know that by adapting to a new energy landscape with battery storage, microgrids, and renewable power sources, and by adopting new cooling technologies, data centers can continue to reduce their carbon emissions. It is only fitting that AI and machine learning integrations themselves hold the power for further optimizing operations to make data centers more efficient and resilient, especially when power capacity shortages plague the industry at large.
I had the fortunate opportunity to discuss these some of these top-of-mind issues on the webinar “Navigating the Sustainable Evolution of Colocation Data Center Design in the AI Era.” Three key areas for reshaping data centers in an AI-first world rose to the surface of that conversation: flexible design, cooling technology advancements, and smaller facility deployments to meet customer sustainability outcomes and performance requirements.
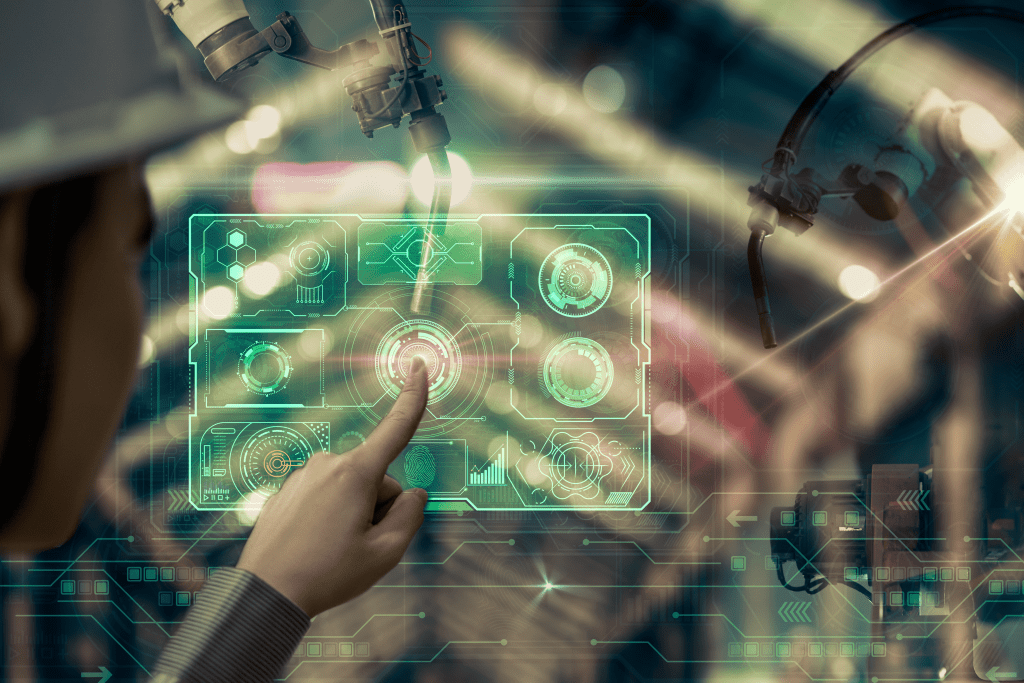
Over the last 20 years, I have witnessed all the nuances and transformations in data center evolution — from rolling out enterprise DCs to shifting to colocation and hyperscale models to adapt to cloud migrations. Today, the industry has reached a new stage with the pressing demands of AI and ML prompting and even accelerating massive research and investments in new AI-ready infrastructure.
Let’s take a closer look at three key areas for ensuring more sustainable deployments in our AI world.
1. Flexible design
Without question, data centers are grappling with the seismic shift in meeting the demands of AI, including generative AI, while operating in a sustainable and responsible way. With AI-ready design capabilities, some colocation facilities already are prepared to handle specialized high-density workloads and face fast-changing data center needs and environments head on.
Partnering with Schneider as an IT and manufacturer specialist can enable colocation service providers to adapt more quickly. What Schneider brings to the table is a decades-long track record of enabling flexible data center design to accommodate changing demand. That means being able to scale up infrastructure quickly (or down, as the case may be) to meet power demands for both higher-density loads and cutting-edge technologies such as liquid cooling. Specifically, Schneider’s flexible, modular infrastructure components can facilitate AI-ready data center transformations.
2. Cooling advancements
IT is evolving right before us. One exciting advancement in the mix is liquid cooling, which is a more efficient way to cool AI and ML loads than air cooling. Being able to accommodate liquid cooling as part of an AI-ready data center design puts colocation providers ahead of the curve. It is important to keep in mind that both liquid cooling and air cooling will be required for the foreseeable future, however. IT equipment is showing a requirement for both with 15-30% of heat rejected to air, and 70-85% removed by liquid cooling.
One of the main implications of liquid cooling is that it requires facilities to accommodate the extra weight for bringing in chilled water. One Schneider customer has figured out how to adjust its data center designs in the most optimal way for AI and ML loads. How? By using space freed up from decommissioned fan wall units. That’s possible because existing facilities are designed for predominately air-cooled IT loads using fan wall units. Fortunately, the transition to cooling distribution units (CDUs) used for liquid cooling does not happen on a one-to-one basis. One CDU can replace between two-four fan walls or crawl units, freeing up space.
A transition to liquid cooling for AI and machine learning loads could result in energy efficiency gains. Rejecting heat to water is more efficient than rejecting it to air. What’s more, fewer cooling units can be used — regardless of whether chillers can be eliminated completely.
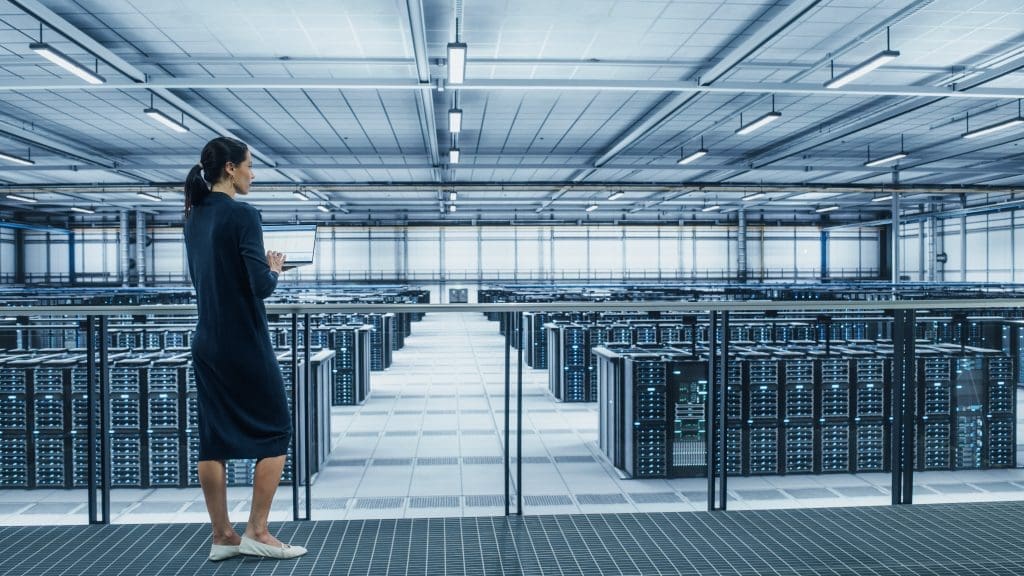
3. Facility size
Efficiency gains are not limited to just liquid cooling. Rack densities for AI and machine learning are becoming much denser — as high as 80 to 100 KW per rack. For instance, I learned from one customer that they can deploy 75 racks in a data center hall designed for AI/ML compared to the 250 or 300 racks needed for a cloud deployment. That means a smaller facility footprint is possible.
This smaller footprint is especially important for addressing Scope 3 emissions, indirect emissions such as embodied carbon in concrete and steel. Higher rack densities translate into smaller data halls and smaller buildings, thereby minimizing construction costs and reducing the carbon footprint by requiring less concrete and steel.
The continued evolution of sustainable data centers in an AI-first world
I am used to managing change throughout the data center industry and the resulting opportunities. The key to navigating that change is starting with a flexible design instead of approaching AI requirements as an afterthought.
Together with customers who are industry leaders, Schneider is meeting new challenges with new opportunities. AI-ready data centers are prompting exciting transformations in the industry. We’re ready.
Add a comment