The implementation of artificial intelligence (AI) in healthcare is significantly impacting how doctors, nurses, and administrators perform their duties. However, the sector is just scratching the surface with AI because the potential number of use cases is immeasurable.
Healthcare and AI use cases
Consider how AI applications are already making a difference — this technology plays a role in robotics, remote surgical assistance, diagnostics, imaging, X-ray analysis, and remote patient monitoring. Increasingly, medical professionals and researchers are applying AI algorithms to analyze patterns in patient data to predict disease and accelerate the development of effective treatments. Possible outcomes are limited only by our imaginations.
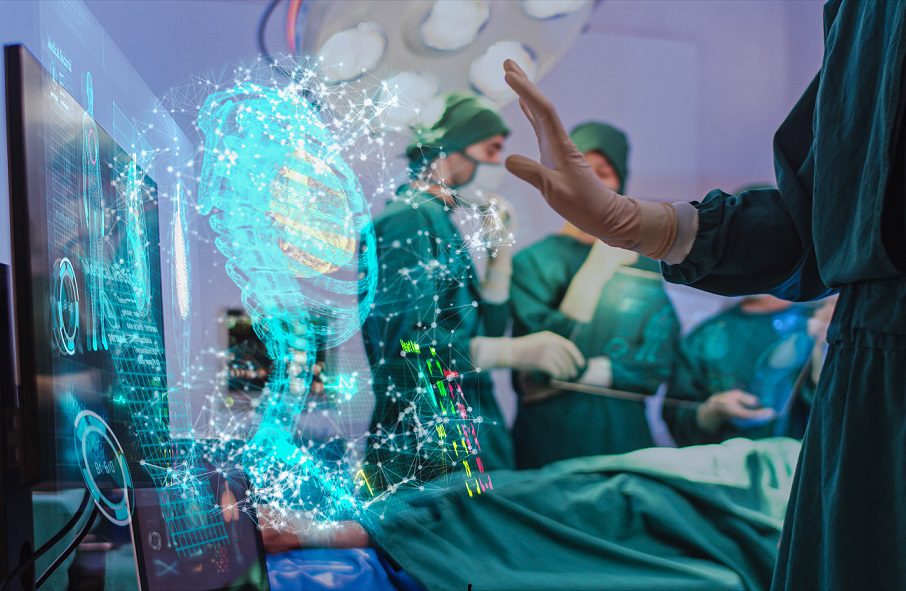
It’s clear AI will have a profound transformative impact on the sector. There is a sense of urgency for healthcare organizations and medical research facilities to ensure they have the necessary IT infrastructure to support advances in the field. Organizations often leverage hyperscale data centers to handle data processed by AI applications, but the need for edge computing sites is also growing.
AI adoption drives explosive data growth
Hospitals produce about 50 petabytes of data yearly, and the healthcare industry accounts for 30% of the world’s data volume. By 2025, the compound annual growth rate of healthcare data will reach 36%, outpacing manufacturing, financial services, and media and entertainment.
AI applications consume and generate massive volumes of data. As more newly trained models are transitioned into production, the number of inference loads or computational workloads will keep growing. This increase in inference loads causes data volumes to explode, boosting the need for additional server capacity and power. In the healthcare sector, added data volume translates to an acute need for flexible, scalable, and available IT infrastructure to manage all the data generated by AI-driven solutions.
For example, let’s look at how an AI training module would function in detecting skin cancer. Dermatologists collect thousands of data sets on skin lesion images with corresponding diagnoses on benign versus malignant samples. The data is then encoded for machine learning analysis and model training is conducted to detect patterns in samples to differentiate between benign and malignant samples. Once the data is validated, the trained module will help dermatologists determine diagnosis, aiding in the early detection and treatment of skin cancer. This is one example of the many AI use cases in healthcare that will rapidly drive the need to analyze data sets.
AI applications can also transform how healthcare providers interpret MRI images, X-rays, and CT scans. Thanks to AI, data can be shared rapidly and remotely for a quicker diagnosis. Telemedicine integrates AI for patient monitoring, intelligent diagnosis, and other purposes. Meanwhile, research labs use AI to analyze vast data sets to find patterns and insights, which can lead to more effective treatments and medications.
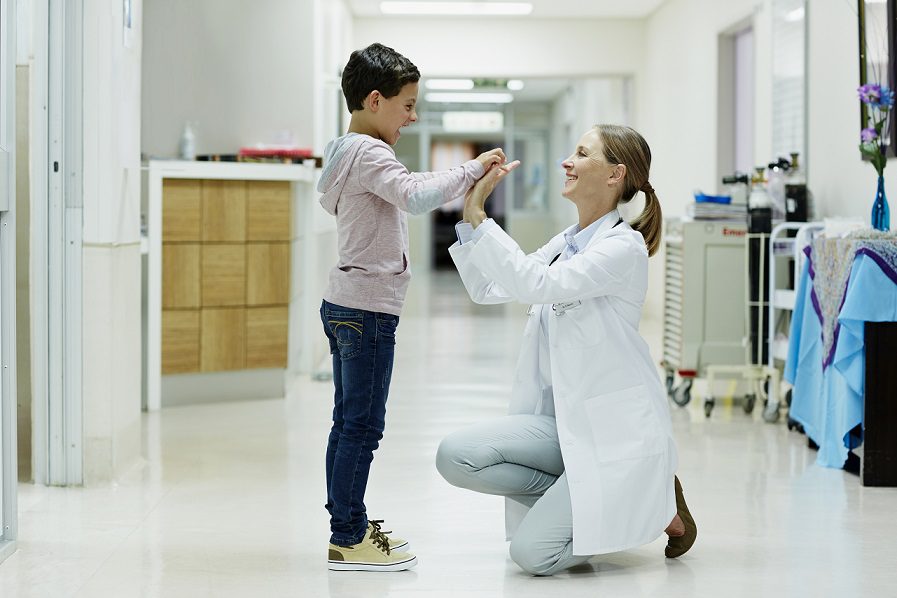
Staffing shortages are another reason for the urgent adoption of AI in healthcare. It’s become increasingly difficult for hospitals to hire and retain nurses. Healthcare organizations in Europe and the United States are struggling to fill vacancies. Some have turned to AI-powered solutions to automate and simplify routine nursing tasks, such as monitoring bedside devices and updating medical charts, enabling nurses to focus more on patient care.
Modernize IT infrastructure to enable AI
The fast data growth rate requires flexible and resilient IT infrastructure so healthcare organizations can quickly adapt to new AI capabilities. This infrastructure can combine centralized clouds and data centers with distributed resources on the edge. Edge computing sites and micro data centers handle data close to the source for real-time applications such as remote patient monitoring and surgical assistance. These use cases require reliable, redundant connectivity.
Healthcare organizations must take decisive steps to prepare and modernize their IT infrastructure. Scalable IT infrastructure, including cooling, power, distribution units, racks, and software, is crucial to support data-intensive AI applications. The sooner healthcare organizations can do this, the sooner they can start to reap the benefits of AI, which ultimately boil down to providing the best patient care possible.
A partner in updating IT infrastructure
As healthcare organizations invest in IT physical infrastructure to support AI, Schneider Electric can guide them through assessment, implementation, and management. Schneider Electric has the experience and solutions to work with healthcare organizations and data center operators to build the necessary IT physical infrastructure: including modular data centers that simplify and accelerate edge computing deployments. Schneider provides solutions for centralized, regional, and edge data center solutions, assisting in retrofitting, modernizing, new designs and builds.
We also offer a comprehensive suite of software and monitoring services, including EcoStruxureTM IT, a vendor-neutral data center infrastructure management (DCIM) solution that offers remote monitoring and management. Healthcare organizations can also leverage our EcoStruxure Advisor solution for modeling single IT racks to hyperscale IT, whether on-premises, in the cloud, or at the edge. Our software and services can play a vital role in healthcare organizations, helping to minimize downtime and build resilience.
Lastly, Schneider Electric can also help organizations implement sustainability strategies to reduce their carbon footprint, even as the need for more power and compute capacity increases. Whether you need a centralized data center or a data center at the edge, discover more data center strategies to adapt to growing AI needs. Also, check out our practical solutions for healthcare organizations.
Add a comment